Table Of Content

Plotting the means is a visualize way to inspect the effects that the independent variables have on the dependent variable. In other words, sunlight and watering frequency do not affect plant growth independently. Rather, there is an interaction effect between the two independent variables. For example, imagine that a researcher wants to do an experiment looking at whether sleep deprivation hurts reaction times during a driving test. If she were only to perform the experiment using these variables–the sleep deprivation being the independent variable and the performance on the driving test being the dependent variable–it would be an example of a simple experiment. You can see that the C and D interaction plot the lines are almost parallel and therefore do not indicate interaction effects that are significant.
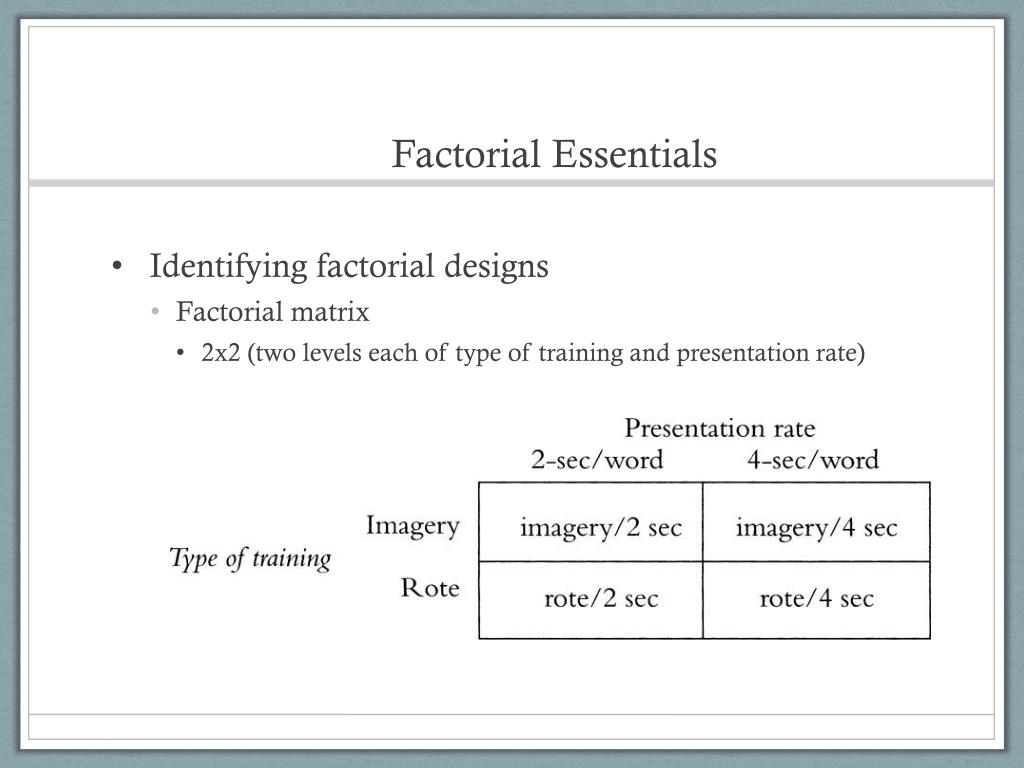
Whether to Use an RCT or a Factorial Design
For instance, in the design depicted in Table 1, the effect of Extended Medication would be reflected by the average effect of all Extended Medication conditions (1–16) versus the average effect of all Standard Medication conditions (17–32). With effect coding, when the experimental conditions have equal (or nearly equal) numbers of participants, the main effect of a factor does not reflect the effects of interaction effects that may be present in the data. Experimentwise error may be more of a problem in factorial designs than in RCTs because multiple main and interactive effects are typically examined. In a 5-factor experiment there are 31 main and interaction effects for a single outcome variable, and more if an outcome is measured at repeated time points and analyzed in a longitudinal model with additional time effects. If more than one outcome variable is used in analyses, the number of models computed and effects tested grow quickly.
Interaction Effects
When there are two independent variables, each with two levels, there are four total conditions that can be tested. It is important to note that interpretation of complex higher order interactions may not be aided by simple effects testing. First, it is highly unlikely that such testing would have distinguished amongst the three leading combinations shown in Figure 1 (the differences in outcomes are too small). Second, such tests would have been grievously underpowered, and increasing the sample size to supply the needed power would have compromised the efficiency of the factorial design (Green et al., 2002). This, of course, has limitations, such as not permitting strong inference regarding the source(s) of the interaction. Alternatively, an investigator might modify an intervention when it co-occurs with a particular, second intervention component.
Minitab Example for Centrifugal Contactor Analysis
The researcher would consider the main effect of sex, the main effect of self-esteem, and the interaction between these two independent variables. Thus far we have seen that factorial experiments can include manipulated independent variables or a combination of manipulated and non-manipulated independent variables. But factorial designs can also include only non-manipulated independent variables, in which case they are no longer experiment designs, but are instead non-experimental in nature.
IV. Chapter 4: Psychological Measurement
Some were negative health-related words (e.g., tumor, coronary), and others were not health related (e.g., election, geometry). The result of this study was that the participants high in hypochondriasis were better than those low in hypochondriasis at recalling the health-related words, but they were no better at recalling the non-health-related words. First, non-manipulated independent variables are usually participant characteristics (private body consciousness, hypochondriasis, self-esteem, and so on), and as such they are, by definition, between-subject factors. For example, people are either low in hypochondriasis or high in hypochondriasis; they cannot be in both of these conditions. Since factorial designs have more than one independent variable, it is also possible to manipulate one independent variable between subjects and another within subjects. For example, a researcher might choose to treat cell phone use as a within-subjects factor by testing the same participants both while using a cell phone and while not using a cell phone.
3.8. Example bar graphs¶
To restate this, in terms of A, the A effect is the difference between the means at the high levels of A versus the low levels of A, whereas the coefficient, \(\alpha_i\), in the model is the difference between the marginal mean and the overall mean. So the Yates "effect" is twice the size of the estimated coefficient αi in the model, which is also usually called the effect of factor A. The table above gives the data with the factors coded for each of the four combinations and below is a plot of the region of experimentation in two dimensions for this case. The quantities b1, b2, and so on are regression weights that indicate how large a contribution an independent variable makes, on average, to the dependent variable. Specifically, they indicate how much the dependent variable changes for each one-unit change in the independent variable. You may find that the patterns of main effects and interaction looks different depending on the visual format of the graph.
Types of Factorial Designs
How To Add Biologics Manufacturing Efficiency With Design Of Experiments Part 2 - BioProcess Online
How To Add Biologics Manufacturing Efficiency With Design Of Experiments Part 2.
Posted: Wed, 05 Apr 2023 07:00:00 GMT [source]
We have usually no knowledge that any one factor will exert its effects independently of all others that can be varied, or that its effects are particularly simply related to variations in these other factors. Typically a three-way interaction would be plotted as two panels... Showing how the two-way interactions differ across the levels of the third factor.
You can reduce this variance by choosing your high and low levels far apart. As we have already seen, researchers conduct correlational studies rather than experiments when they are interested in noncausal relationships or when they are interested variables that cannot be manipulated for practical or ethical reasons. In this section, we look at some approaches to complex correlational research that involve measuring several variables and assessing the relationships among them. The presence of an interaction, particularly a strong interaction, can sometimes make it challenging to interpet main effects. For example, take a look at Figure 5.14, which indicates a very strong interaction. To briefly add to the confusion, or perhaps to illustrate why these two concepts can be confusing, we will look at the eight possible outcomes that could occur in a 2x2 factorial experiment.
You won't make a Type I error, however you could more likely make a Type II error. We have done the hard work of finding an effect of interest, in this case the distraction effect. We think this distraction effect actually measures something about your ability to pay attention. If we think that we found an effect of distraction, maybe we could find a way to reduce distraction to help students focus on their homework? Or help my partner focus on the intricate plot of TV show that we're watching?
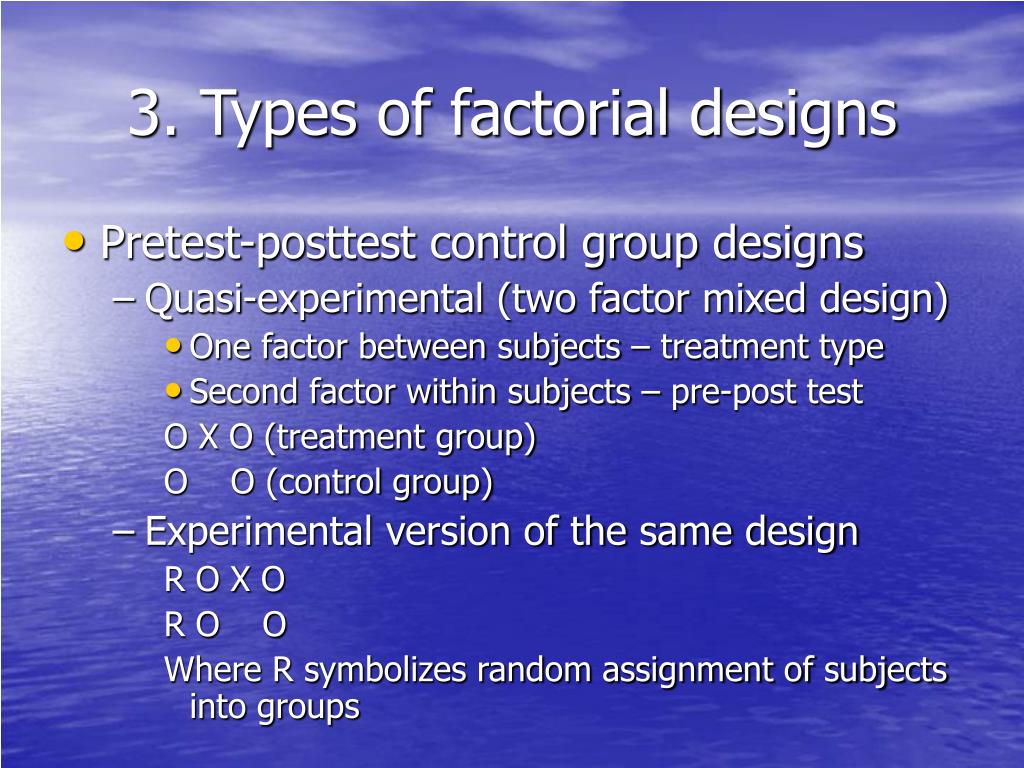
After all, only a small portion of a sample in a factorial experiment will get a particular set of components (e.g., in the design depicted in Table 1 only 1/32 of the N will get a particular combination of components). In many factorial designs, one of the independent variables is a non-manipulated independent variable. One independent variable was disgust, which the researchers manipulated by testing participants in a clean room or a messy room. The other was private body consciousness, a variable which the researchers simply measured.
However, conducting an RCT that comprises ICs whose joint effects are unknown, poses clear risks. This is because research shows that the effectiveness of a IC can be substantially modulated by the other ICs with which it is used (Cook et al., 2016; Fraser et al., 2014; Schlam et al., 2016); i.e., they may interact. An example is a study by Halle Brown and colleagues in which participants were exposed to several words that they were later asked to recall (Brown, Kosslyn, Delamater, Fama, & Barsky, 1999)[1]. Some were negative health-related words (e.g., tumor, coronary), and others were not health related (e.g., election, geometry). For example, a researcher might choose to treat cell phone use as a within-subjects factor by testing the same participants both while using a cell phone and while not using a cell phone (while counterbalancing the order of these two conditions).
Examples of these plots can be found in the Minitab Example for Centrifugal Contactor Analysis. The alpha value, which determines the limit of statistical significance, can be chosen in this menu also. A common one to select is "Residuals versus fits" which shows how the variance between the predicted values from the model and the actual values.
No comments:
Post a Comment